In the past decade, artificial intelligence has made significant advancements in the field of generative models. These models have the ability to create new data that resembles the training data it was fed. With the help of video generative models, AI developers can now create videos that are indistinguishable from real-life footage. In this article, we'll explore the latest advancements in generative AI development and how video generative models are revolutionizing the field.
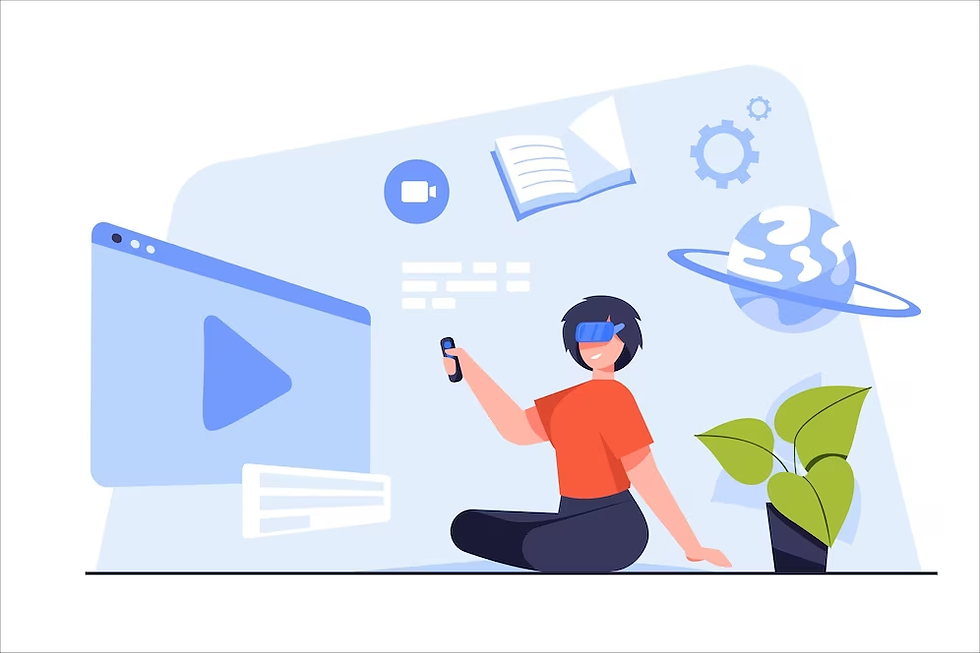
What are Generative Models?
Generative models are a type of machine learning algorithm that can generate new data based on the training data it was given. These models have the ability to learn the underlying structure of the data and use it to generate new examples that resemble the training data. For example, if you train a generative model on a dataset of cat images, it can generate new images of cats that look like the ones in the training dataset.
Generative models have a wide range of applications, including image and text generation, style transfer, and anomaly detection. With the help of deep learning, generative models have become more powerful and efficient in recent years.
What are Video Generative Models?
Video generative models are a type of generative model that can generate videos that look like real-life footage. These models use a technique called "temporal convolution" to generate video frames that are consistent with the frames that came before them. Video generative models are trained on large datasets of video footage, such as movies or TV shows. The model learns the patterns and structures of the video frames and uses this information to generate new frames that resemble the original footage.
Applications of Video Generative Models
Video generative models have a wide range of applications in various industries, including entertainment, gaming, and virtual reality. For example, video generative models can be used to create realistic animations for movies or video games. They can also be used to create virtual reality environments that look and feel like real-life. Another application of video generative models is in the field of surveillance. With the help of these models, security cameras can be trained to generate video footage of potential suspects, even if they were not captured on camera. This can be a valuable tool for law enforcement agencies in identifying and tracking down criminals.
Advancements in Generative AI Development with Video Generative Models
In recent years, there have been significant advancements in generative AI development with the help of video generative models. One of the most significant advancements is the use of Generative Adversarial Networks (GANs). GANs are a type of generative model that consists of two neural networks: a generator and a discriminator. The generator creates new data, while the discriminator evaluates the data and determines whether it is real or fake. The two networks are trained together, with the goal of creating new data that is indistinguishable from the real data. GANs have been used to create some of the most impressive video generative models to date. For example, researchers at NVIDIA have created a GAN-based video generative model that can generate realistic videos of city streets. The model was trained on a dataset of 24,000 videos and can generate new videos that are difficult to distinguish from real-life footage. Another significant advancement in generative AI development with video generative models is the use of attention mechanisms. Attention mechanisms allow the model to focus on specific parts of the video frame when generating new frames. This results in more realistic and detailed videos.
Conclusion
In conclusion, generative models have come a long way in recent years, and video generative models are leading the way in generative AI development. With the help of GANs and attention mechanisms, video generative models can now create videos that are almost indistinguishable from real-life footage. These models have a wide range of applications in various industries, including entertainment, gaming, and security.
As generative AI development continues to advance, we can expect to see even more impressive video generative models in the future. These models have the potential to revolutionize the way we create and consume media, and they may even lead to new applications that we have not yet imagined.
However, as with any technology, there are also potential ethical concerns associated with video generative models. For example, these models could be used to create deepfake videos that could be used to spread false information or even blackmail individuals.
Therefore, it is important for AI developers and policymakers to consider the potential implications of this technology and to take steps to ensure that it is used in a responsible and ethical manner.
In conclusion, video generative models are a promising advancement in the field of generative AI development. With the help of GANs and attention mechanisms, these models have the ability to create videos that are almost indistinguishable from real-life footage. While there are potential ethical concerns associated with this technology, it is important to continue to explore its potential applications and to use it in a responsible and ethical manner.
Comments