Introduction:
In the rapidly evolving world of artificial intelligence (AI), Explainable AI (XAI) has emerged as a crucial concept. As AI systems become more complex and sophisticated, understanding how they make decisions is essential for gaining trust, ensuring accountability, and addressing potential biases. In this article, we will delve into the concept of Explainable AI and explore its significance in the realm of machine learning algorithms.
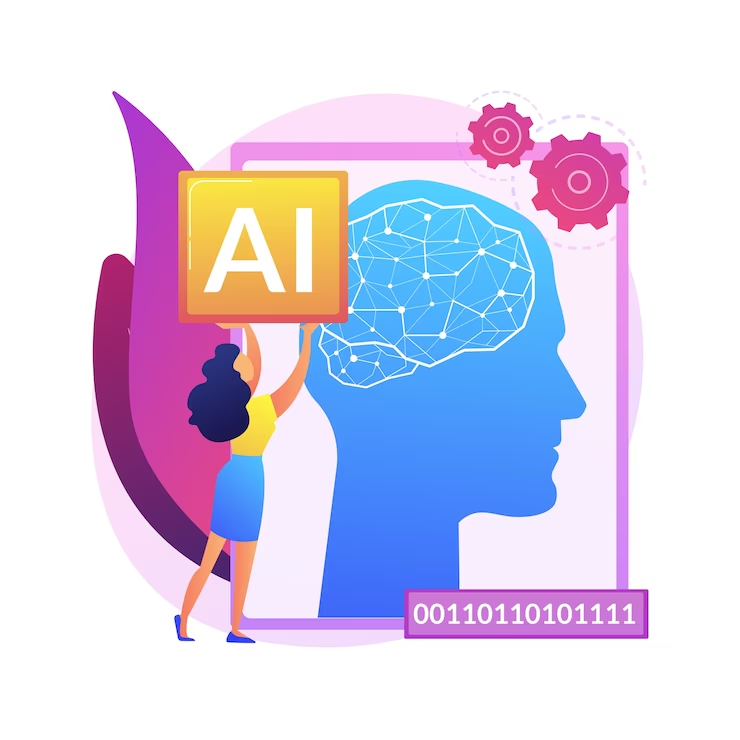
Understanding Explainable AI:
Explainable AI refers to the ability of an AI system to provide clear and understandable explanations for its decision-making processes. It allows users, developers, and stakeholders to comprehend why an AI model arrived at a specific outcome, enabling them to identify and rectify any errors or biases. Unlike traditional black-box AI models, Explainable AI provides transparency and interpretability, bridging the gap between human understanding and machine learning.
The Need for Explainable AI:
1. Trust and Accountability: In domains where AI impacts critical decision-making, such as healthcare, finance, and law enforcement, trust is paramount. Stakeholders need to trust AI systems to make fair, unbiased, and reliable decisions. Explainable AI helps establish trust by shedding light on the reasoning behind AI-generated outcomes.
2. Bias Detection and Mitigation: Biases within AI models can perpetuate societal inequalities. Explainable AI allows for the identification and mitigation of biased decision-making, ensuring that AI systems operate in a fair and just manner. By uncovering discriminatory patterns, stakeholders can take corrective actions to enhance the inclusivity of AI applications.
3. Regulatory Compliance: With the increasing focus on data privacy and ethical considerations, regulations surrounding AI are becoming more stringent. Explainable AI plays a crucial role in meeting these requirements, as it allows auditors and regulators to understand the inner workings of AI models and ensure compliance with legal and ethical guidelines.
Methods and Techniques for Explainable AI:
1. Model Transparency: Developing AI models with built-in transparency is the first step towards explainability. Techniques like decision trees, rule-based models, and linear regression models inherently provide interpretable outputs, making them suitable for Explainable AI applications.
2. Post-hoc Explainability: For existing black-box AI models, post-hoc explainability techniques can be employed. These techniques involve extracting insights from the model's internal mechanisms, such as feature importance, activation maps, or attention weights. Methods like LIME (Local Interpretable Model-agnostic Explanations) and SHAP (SHapley Additive exPlanations) are popular in this domain.
3. Rule Extraction: Rule extraction methods aim to extract human-readable rules from complex AI models. These rules provide explanations for model predictions, allowing stakeholders to understand the decision-making process. Techniques like symbolic rule extraction and decision rule induction are commonly used for this purpose.
4. Interactive Visualization: Visualization techniques help present complex AI models and their decisions in a visually intuitive manner. Interactive visualizations enable users to explore and understand the model's behavior, fostering a deeper comprehension of AI outcomes.
Benefits and Future Implications:
Embracing Explainable AI brings several benefits to diverse domains, such as healthcare diagnostics, credit scoring, autonomous vehicles, and fraud detection. By understanding the logic behind AI systems, stakeholders can identify system weaknesses, improve model performance, and ensure fair and unbiased decision-making.
However, the journey towards widespread adoption of Explainable AI is not without challenges. Striking a balance between explainability and performance remains a significant concern. Researchers and practitioners are continually striving to develop techniques that maintain high accuracy while providing understandable explanations.
Conclusion:
Explainable AI is transforming the AI landscape by offering transparency, trust, and accountability. As AI becomes increasingly embedded in our lives, understanding and interpreting its decisions are paramount. By embracing Explainable AI, we can unlock the true potential of AI systems while ensuring they align with ethical and societal values. Let's work towards a future where AI is not just intelligent but also explainable.
Comments