Ensuring Robust Data Security in AI Systems: A Comprehensive Guide
- Christopher T. Hyatt
- Jun 20, 2023
- 2 min read
Introduction:
In an era driven by artificial intelligence (AI), data security has become a paramount concern. The proliferation of AI systems has led to an exponential increase in the amount of data being generated and processed. As organizations leverage the power of AI to unlock valuable insights, it becomes imperative to address the challenges and risks associated with data security. In this article, we will explore the importance of data security in AI systems and discuss effective strategies to ensure robust protection.
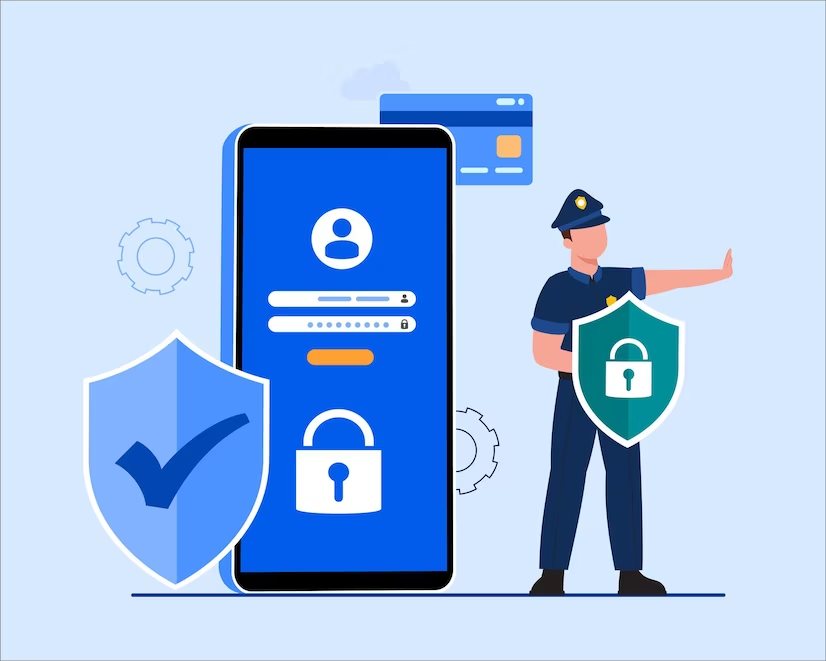
Understanding the Significance of Data Security in AI Systems:
Data security forms the bedrock of trustworthy AI systems. With the advent of sophisticated algorithms and machine learning models, vast amounts of sensitive information are processed, including personal, financial, and proprietary data. Ensuring the confidentiality, integrity, and availability of this data is crucial to prevent unauthorized access, data breaches, and potential misuse. AI systems that handle sensitive information must adhere to stringent data security measures to foster user confidence and compliance with regulatory requirements.
Key Challenges and Risks in Data Security for AI Systems:
1. Data Breaches: AI systems are attractive targets for cybercriminals due to the valuable insights and sensitive information they hold. A data breach can have severe consequences, including financial loss, reputational damage, and legal repercussions. Protecting against unauthorized access, both externally and internally, is essential.
2. Adversarial Attacks: AI systems are susceptible to adversarial attacks, where malicious actors exploit vulnerabilities to manipulate the system's output. This can have far-reaching consequences, such as biased decision-making, compromised user privacy, and erroneous results. Robust data security measures should include defenses against adversarial attacks.
3. Insider Threats: Organizations must also consider the risk of insider threats, where authorized individuals with access to AI systems misuse or leak sensitive data. Implementing access controls, monitoring mechanisms, and proper employee training can help mitigate this risk.
Strategies for Ensuring Data Security in AI Systems:
1. Encryption: Implement strong encryption techniques to protect data at rest and in transit. Encryption ensures that even if unauthorized access occurs, the data remains unreadable and unusable.
2. Access Controls: Employ strict access controls to limit system access to authorized individuals only. Use strong authentication mechanisms such as multi-factor authentication and role-based access control (RBAC) to prevent unauthorized entry.
3. Regular Auditing and Monitoring: Implement robust auditing and monitoring systems to track system activity, detect anomalies, and promptly respond to potential security breaches. Continuous monitoring helps identify and mitigate security risks in real-time.
4. Privacy-Preserving Techniques: Utilize privacy-preserving techniques such as differential privacy, federated learning, and homomorphic encryption to protect sensitive data while still allowing for effective analysis and model training.
5. Regular Patching and Updates: Keep AI systems and associated software up to date with the latest security patches. Regularly apply updates and security fixes to address vulnerabilities and protect against emerging threats.
Conclusion:
Data security in AI systems is a critical concern that requires proactive measures to mitigate risks and protect sensitive information. By implementing robust encryption, access controls, auditing, and monitoring mechanisms, organizations can ensure the confidentiality, integrity, and availability of data. Furthermore, the use of privacy-preserving techniques and staying updated with security patches enhances the resilience of AI systems. Prioritizing data security in AI systems is not only essential for compliance with regulations but also for building trust and maintaining a competitive edge in today's data-driven landscape.
Comments