Introduction:
In today's data-driven world, machine learning has become a powerful tool for extracting insights and making informed decisions. However, developing accurate and efficient machine learning models traditionally requires extensive expertise and time-consuming manual processes. This is where AutoML (Automated Machine Learning) steps in, revolutionizing the field by automating and simplifying the model development process. In this article, we will explore the world of AutoML and its transformative impact on businesses, while shedding light on the benefits and key considerations when implementing this cutting-edge technology.
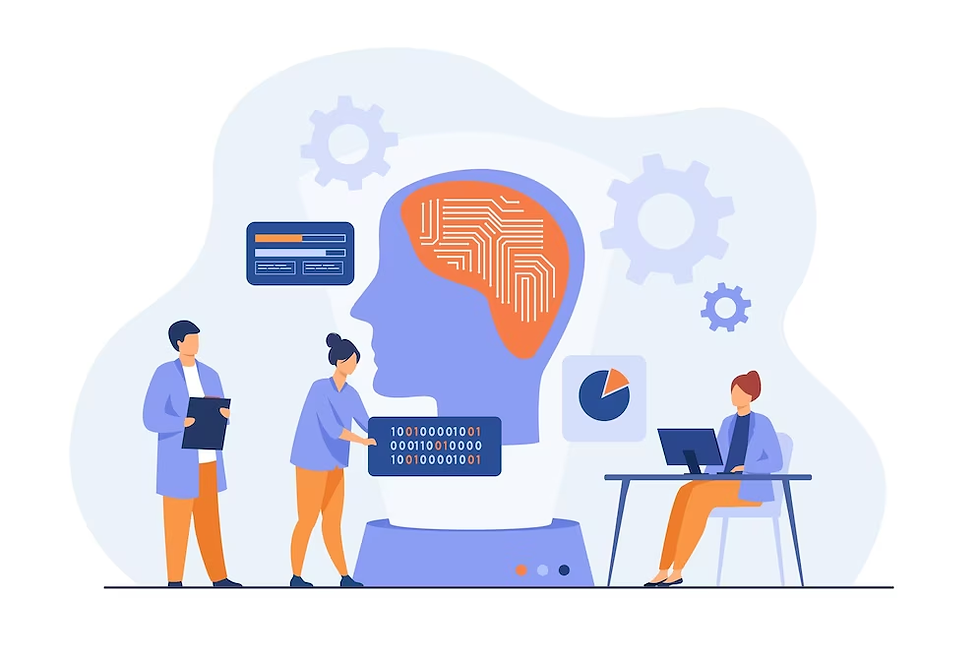
Understanding AutoML:
AutoML refers to a collection of automated techniques that enable organizations to build and deploy machine learning models without extensive programming or data science expertise. It leverages artificial intelligence and machine learning algorithms to automate various stages of the model development pipeline, including data preprocessing, feature engineering, model selection, and hyperparameter tuning. By automating these complex tasks, AutoML empowers even non-experts to harness the power of machine learning, driving faster time-to-insights and democratizing the field.
Benefits of AutoML:
1. Increased Efficiency: AutoML eliminates the need for manual trial and error, significantly reducing the time and effort required to develop high-performing models. This allows data scientists and analysts to focus on more strategic tasks, such as interpreting and applying the insights generated by the models.
2. Democratization of Machine Learning: With AutoML, organizations can enable individuals without extensive coding knowledge to leverage machine learning. This expands the talent pool and empowers domain experts to build models tailored to their specific needs, unlocking new opportunities for innovation and decision-making.
3. Improved Accuracy: AutoML algorithms explore a wide range of model architectures, feature combinations, and hyperparameter settings to identify the optimal configuration for a given problem. This systematic approach often leads to improved model accuracy and generalization, enhancing the quality of predictions and insights.
Key Considerations for Implementing AutoML:
1. Quality and Diversity of Data: AutoML's performance heavily relies on the quality, quantity, and diversity of the training data. Ensuring a well-curated and representative dataset is essential to achieve accurate and reliable models.
2. Interpretability and Explainability: While AutoML automates the model development process, it's important to assess the interpretability and explainability of the resulting models. Understanding how the model makes predictions is crucial for gaining trust and addressing regulatory and ethical concerns.
3. Scalability and Deployment: When choosing an AutoML solution, it's essential to consider its scalability and deployment capabilities. Ensuring the platform can handle large datasets, integrates with existing infrastructure, and supports easy deployment to production environments will maximize the value derived from AutoML.
4. Continuous Monitoring and Model Maintenance: Machine learning models require ongoing monitoring and maintenance to ensure their performance remains optimal over time. Regularly retraining models, updating data, and monitoring for performance drift are critical steps in the AutoML lifecycle.
Conclusion:
AutoML has emerged as a game-changer in the field of machine learning, allowing organizations to unlock the power of data-driven decision-making without the need for extensive coding or data science expertise. By automating the model development pipeline, AutoML accelerates time-to-insights, democratizes machine learning, and enhances model accuracy. However, successful implementation of AutoML requires careful consideration of data quality, interpretability, scalability, and ongoing maintenance. By harnessing the transformative potential of AutoML, businesses can gain a competitive edge, drive innovation, and make smarter, data-driven decisions in today's fast-paced digital landscape.
Comments