Introduction:
Reinforcement learning, a subfield of artificial intelligence (AI), has made significant strides in recent years. One promising approach within this domain is reinforcement learning from human feedback. This article explores the potential of leveraging human knowledge to enhance the performance of reinforcement learning algorithms. Discover how this technique can revolutionize AI advancements and drive intelligent decision-making processes.]
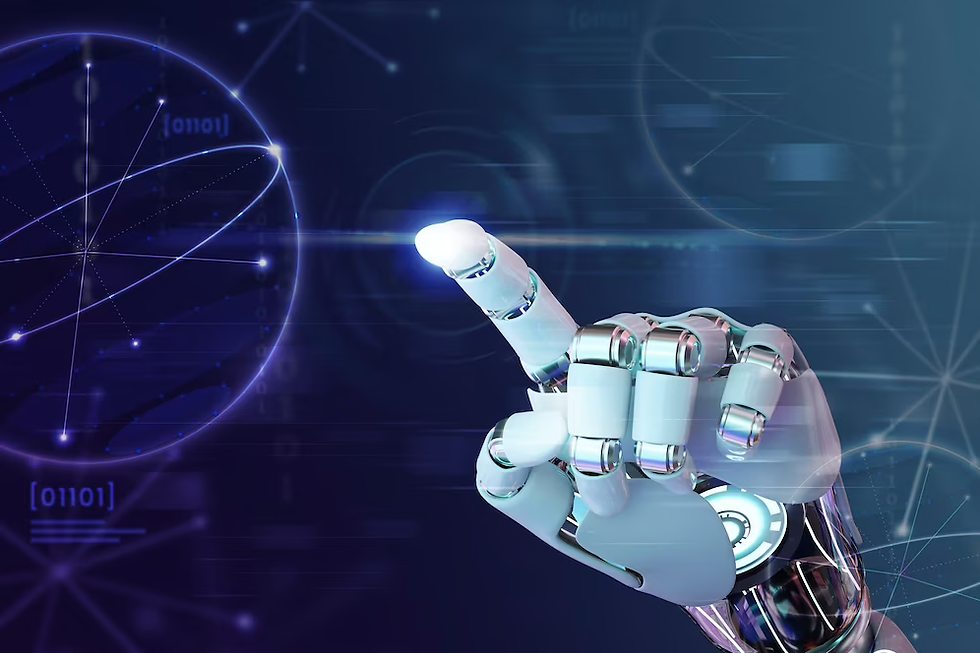
Understanding Reinforcement Learning from Human Feedback:
Reinforcement learning from human feedback involves training AI models by incorporating insights and guidance from human experts. By harnessing the power of human expertise, AI algorithms can improve their decision-making capabilities and adapt more effectively to complex environments.
Benefits and Applications:
This approach offers several benefits across various domains. Firstly, it enables faster and more efficient training of reinforcement learning models, reducing the time and resources required. Secondly, by utilizing human feedback, these models can gain insights and knowledge that may be difficult to acquire solely through trial and error. This makes reinforcement learning from human feedback particularly valuable in scenarios where expert guidance is readily available.
The applications of reinforcement learning from human feedback are vast. In autonomous vehicles, human expertise can be used to fine-tune driving behaviors, leading to safer and more reliable self-driving cars. In robotics, human feedback can guide robots to perform complex tasks with precision and adaptability. Additionally, this technique can be applied to optimize resource allocation, enhance recommender systems, and improve healthcare decision-making.
Techniques for Leveraging Human Feedback:
Two key techniques for reinforcement learning from human feedback are reward modeling and inverse reinforcement learning. Reward modeling involves constructing an appropriate reward function based on human preferences or demonstrations. This approach allows AI models to learn desired behavior more effectively. Inverse reinforcement learning, on the other hand, focuses on inferring the underlying reward function from observed human behavior. This enables AI models to understand the intentions and objectives of human experts, leading to more accurate decision-making.
Challenges and Future Directions:
While reinforcement learning from human feedback holds immense potential, it also presents certain challenges. Obtaining high-quality and diverse human feedback is essential for effective training. Balancing the trade-off between exploration and exploitation is another challenge that researchers and developers need to address.
In the future, advancements in reinforcement learning from human feedback will likely involve integrating techniques like imitation learning and interactive learning, further augmenting AI capabilities. Researchers will continue to refine algorithms to handle noisy or inconsistent human feedback, enabling more robust and reliable AI systems.
Conclusion:
Reinforcement learning from human feedback represents a significant milestone in the development of AI systems. By harnessing human expertise, we can unlock the full potential of reinforcement learning algorithms and enhance their performance across various domains. As advancements continue, we can expect to witness groundbreaking applications of this approach, revolutionizing industries and driving intelligent decision-making processes. Embracing reinforcement learning from human feedback is a step toward creating AI systems that truly understand and learn from human knowledge.
Read more
Comments