Introduction:
In today's data-driven world, businesses are relying on data analysis to gain valuable insights and make informed decisions. However, with the increasing volume and complexity of data, it's crucial to have an efficient data analysis workflow in place. In this article, we will delve into the key components of a data analysis workflow and explore how to optimize it for maximum productivity and accuracy.
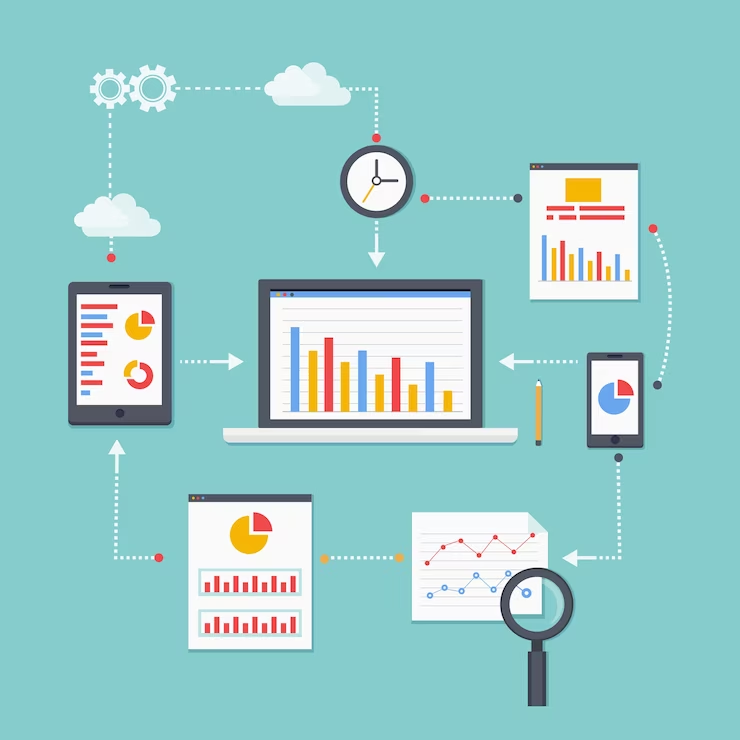
Understanding Data Analysis Workflow
In this section, we will explain the concept of a data analysis workflow and its significance in the decision-making process. We'll discuss the stages involved, such as data collection, data cleaning, exploratory analysis, modeling, and result interpretation. By providing a clear overview, readers will grasp the importance of a well-structured workflow.
Data Collection and Preprocessing
Data collection is the foundation of any data analysis project. We will explore various sources and techniques for collecting data, including web scraping, API integration, and data repositories. Furthermore, we will discuss the importance of data preprocessing, which involves handling missing values, outlier detection, and feature engineering. By optimizing this stage, analysts can ensure high-quality data for accurate analysis.
Exploratory Data Analysis (EDA)
EDA plays a vital role in understanding the underlying patterns and relationships within a dataset. We will explore popular techniques for visualizing and summarizing data, such as histograms, scatter plots, and correlation matrices. Additionally, we will discuss how to identify trends, detect anomalies, and extract meaningful insights from the data.
Modeling and Analysis Techniques
This section will focus on different modeling and analysis techniques commonly used in data analysis workflows. We will cover regression, classification, clustering, and time series analysis, explaining their applications and benefits. Furthermore, we will discuss how to evaluate model performance and make data-driven decisions based on the analysis results.
Result Interpretation and Reporting
The final stage of a data analysis workflow is interpreting the results and effectively communicating them to stakeholders. We will explore techniques for visualizing and presenting data analysis findings in a clear and compelling manner. Additionally, we will emphasize the importance of storytelling and data-driven narratives to convey the insights effectively.
Conclusion:
A streamlined data analysis workflow is the backbone of successful data-driven decision-making. By following a well-defined process that encompasses data collection, preprocessing, exploratory analysis, modeling, and result interpretation, businesses can unlock the true potential of their data. Remember, the key lies in leveraging the right tools, techniques, and best practices to extract meaningful insights and drive positive outcomes.
By optimizing your data analysis workflow, you can gain a competitive edge in today's data-centric world and make informed decisions that propel your business forward.
Comments