Demystifying AI: The Principles of Explainable AIDemystifying AI: The Principles of Explainable AI
- Christopher T. Hyatt
- Jul 25, 2023
- 3 min read
Introduction
Artificial Intelligence (AI) has witnessed remarkable progress over the years, revolutionizing various industries and impacting our daily lives. As AI systems become increasingly complex and powerful, the need for understanding their decision-making processes has grown significantly. Enter Explainable AI (XAI), a critical area of research focused on making AI models more transparent and interpretable. In this article, we will explore the principles of Explainable AI and its importance in ensuring the responsible and ethical use of AI technologies.
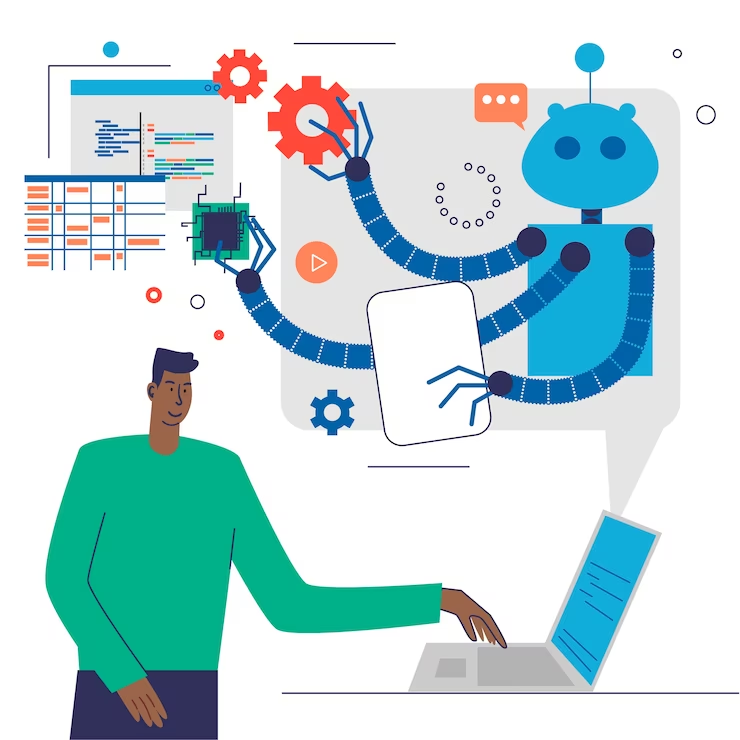
1. Transparency
The first and foremost principle of Explainable AI is transparency. An AI system should be able to provide a clear and coherent explanation of how it arrived at a particular decision or prediction. This involves revealing the underlying mechanisms, data, and features that influenced the AI model's output. Transparent AI not only builds trust among users but also helps experts and stakeholders to identify potential biases and errors, making the model more robust and reliable.
2. Human-Interpretable Representation
Explainable AI systems aim to present information in a manner that humans can easily comprehend. Using technical jargon or complex mathematical models can hinder understanding, defeating the purpose of XAI. Therefore, AI researchers and developers strive to create human-interpretable representations of the AI model's behavior, such as feature importance scores, decision trees, or natural language explanations.
3. Local vs. Global Explanations
Explainable AI can offer both local and global explanations. Local explanations focus on explaining the predictions of a specific instance or data point, while global explanations provide insights into the model's overall behavior. A combination of both is often necessary to gain a comprehensive understanding of an AI system's strengths and limitations.
4. Model Simplicity
Simplicity is a valuable principle in building explainable AI models. While complex AI architectures might achieve state-of-the-art performance, they can be challenging to explain. Simpler models, such as linear models or decision trees, are often preferred in XAI because they provide clear and intuitive explanations.
5. Incorporating Human Feedback
Explainable AI is not solely about technical implementations; it also involves incorporating human feedback into the process. By actively involving end-users, domain experts, and other stakeholders, AI systems can learn from human insights and refine their explanations over time. Human feedback is instrumental in identifying and rectifying potential biases, ensuring fair and ethical AI applications.
6. Context and Task-Specific Explanations
The interpretability of AI models heavily depends on the context and the task they are designed to perform. Different applications might require different forms of explanations. For instance, in medical AI, it is crucial to provide detailed explanations for diagnostic decisions, whereas in natural language processing, understanding how language embeddings influence predictions might be more relevant.
7. Trade-off between Performance and Explainability
A crucial challenge in Explainable AI is finding the right balance between model performance and explainability. Highly interpretable models might sacrifice predictive accuracy, while complex models may compromise on transparency. Striking a balance requires careful consideration of the specific use case and the desired level of interpretability.
Conclusion
Explainable AI principles are indispensable in shaping the future of artificial intelligence, making it more transparent, understandable, and accountable. As AI continues to integrate into various aspects of our lives, ensuring that these technologies can be explained and justified becomes imperative. Transparent AI models not only promote user trust but also enable AI to be deployed responsibly, addressing concerns about bias, discrimination, and the "black box" problem. By adhering to the principles of Explainable AI, we can unlock the full potential of AI while safeguarding human values and ethical standards.
Comments