Introduction:
In the realm of Artificial Intelligence (AI) and Natural Language Processing (NLP), prompt engineering has emerged as a powerful technique to enhance the performance and control the behavior of AI language models. As the field of AI continues to advance rapidly, it becomes crucial to understand and leverage prompt engineering to optimize the output of AI systems. This article aims to provide a comprehensive overview of what is prompt engineering, its significance, and practical applications.
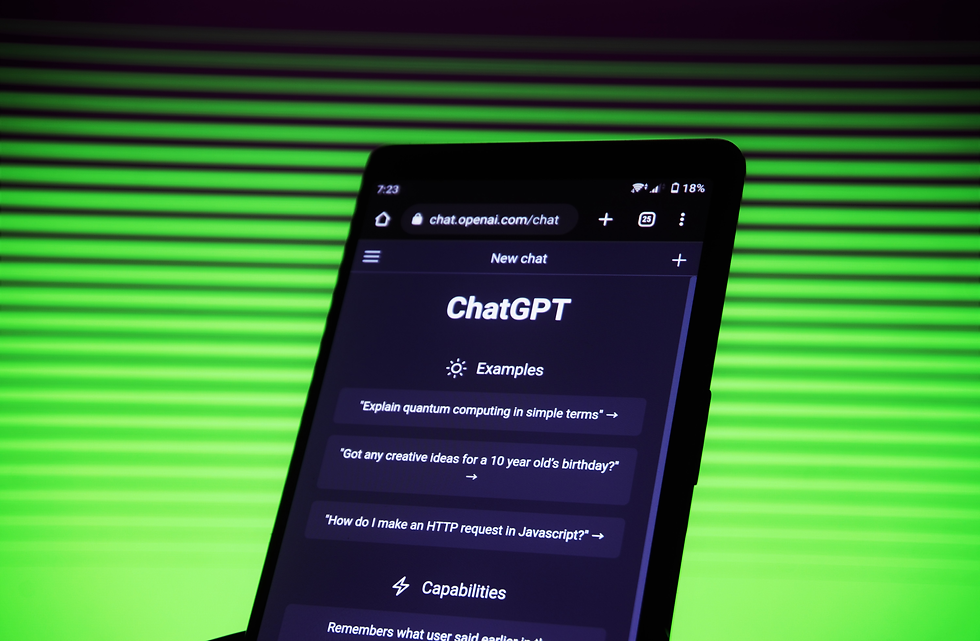
What is Prompt Engineering?
Prompt engineering involves the design and formulation of precise instructions or prompts to guide AI language models towards desired outputs. It enables users to specify the desired behavior or context for the model, shaping its responses accordingly. By providing a structured input, prompt engineering enables users to obtain specific and relevant results, making AI language models more reliable and useful.
The Significance of Prompt Engineering:
Prompt engineering plays a vital role in fine-tuning the behavior of AI language models. Without proper guidance, these models may generate biased or untrustworthy outputs. By employing prompt engineering techniques, researchers and developers can mitigate biases, improve accuracy, and ensure the generation of contextually appropriate responses. Additionally, prompt engineering allows users to customize the AI model's responses based on their specific requirements, making it a versatile tool across various domains.
Applications of Prompt Engineering:
1. Question Answering Systems: Prompt engineering enables the development of question-answering systems that can accurately provide information based on specific prompts. By crafting well-designed prompts, developers can enhance the model's ability to retrieve relevant and reliable information from vast knowledge bases.
2. Text Completion and Generation: With prompt engineering, AI language models can generate coherent and contextually appropriate text. This application is particularly useful for content creation, where prompts can guide the model to produce engaging and informative articles, blog posts, and social media updates.
3. Sentiment Analysis and Review Generation: Prompt engineering allows users to train AI models to analyze sentiments and generate reviews or feedback based on specific guidelines. This application finds utility in product reviews, customer feedback analysis, and sentiment classification tasks.
4. Translation and Summarization: By utilizing prompt engineering, AI language models can be fine-tuned to perform accurate translation and summarization tasks. This ensures better quality translations and concise summaries based on user-specified instructions.
Best Practices for Effective Prompt Engineering:
To maximize the benefits of prompt engineering, consider the following best practices:
1. Clearly define the desired output: Formulate prompts that precisely specify the desired information, context, or behavior you want the AI model to generate.
2. Experiment with different prompts: Iterate and test various prompts to observe how they impact the model's output. Fine-tuning and refining the prompts can significantly improve the results.
3. Consider bias mitigation: Pay attention to potential biases in the generated outputs and incorporate prompt engineering techniques to address and minimize biases.
4. Leverage context and constraints: Utilize additional context or constraints within prompts to guide the model's response and ensure it aligns with specific requirements or guidelines.
Conclusion:
Prompt engineering is a powerful technique that empowers users to mold the behavior of AI language models to produce desired outputs. By leveraging prompt engineering, developers and researchers can enhance the accuracy, reliability, and contextuality of AI systems. As AI continues to advance, understanding and applying prompt engineering techniques will become increasingly crucial for optimizing AI language model performance across various domains.
Comments