Introduction:
Machine Learning Operations (MLOps) has emerged as a game-changing practice that combines the power of machine learning with the efficiency of operations. As the demand for intelligent systems grows, organizations are increasingly realizing the importance of implementing MLOps to streamline their machine learning workflows. In this article, we will explore the concept of MLOps and its significance in driving business success. So, let's dive in!
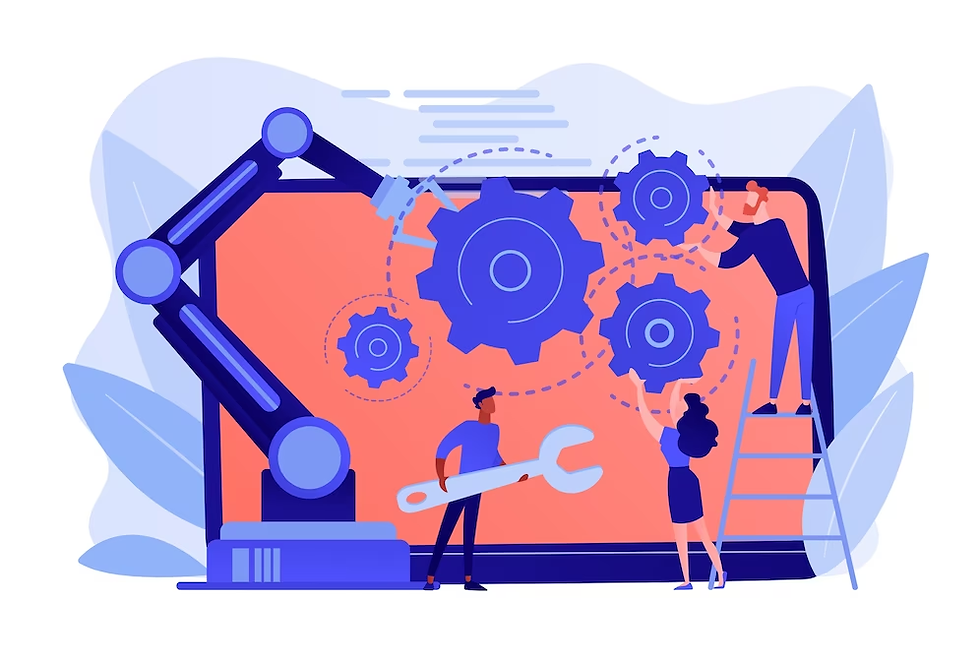
Understanding Machine Learning Operations:
Machine Learning Operations, or MLOps, refers to the set of practices and tools designed to streamline and automate the deployment, management, and monitoring of machine learning models in production environments. It encompasses the entire lifecycle of a machine learning model, from development and training to deployment and ongoing maintenance.
Why Machine Learning Operations Matter:
1. Efficient Model Development: MLOps facilitates collaboration among data scientists, engineers, and other stakeholders, ensuring a smooth transition from experimentation to production. By incorporating version control and automated testing, MLOps helps teams iterate faster and develop robust models.
2. Scalable Model Deployment: With MLOps, organizations can seamlessly deploy machine learning models across different environments, whether on-premises or in the cloud. This scalability enables businesses to leverage the full potential of their models, delivering value at scale and meeting increasing demands.
3. Continuous Monitoring and Improvement: MLOps enables real-time monitoring of deployed models, tracking their performance and detecting anomalies. By proactively identifying issues, organizations can ensure optimal model performance, make timely improvements, and enhance business outcomes.
4. Enhanced Security and Governance: MLOps ensures that machine learning models adhere to regulatory and compliance requirements. It provides features like model versioning, access controls, and audit trails, empowering organizations to maintain data privacy and establish robust governance practices.
Implementing Machine Learning Operations:
1. Automated Pipelines: MLOps relies on automated pipelines to orchestrate the various stages of the machine learning lifecycle. These pipelines automate data preprocessing, model training, and deployment processes, reducing manual effort and improving efficiency.
2. Infrastructure Management: Effective MLOps requires managing the infrastructure necessary to support machine learning workflows. This includes provisioning scalable computing resources, managing containers or virtual machines, and utilizing container orchestration platforms like Kubernetes.
3. Continuous Integration and Delivery (CI/CD): Implementing CI/CD practices in MLOps enables seamless integration of model updates, automated testing, and smooth deployment to production. This ensures a consistent and reliable delivery of machine learning models.
4. Monitoring and Alerting: Real-time monitoring of deployed models is essential for identifying performance issues, data drift, or concept drift. MLOps leverages monitoring tools and techniques to detect anomalies, generate alerts, and trigger automated actions for proactive maintenance.
Conclusion:
Machine Learning Operations (MLOps) has revolutionized the way organizations deploy, manage, and monitor machine learning models. By integrating MLOps practices, businesses can enhance their model development, ensure scalable deployments, continuously improve model performance, and maintain robust security and governance. Embracing MLOps is crucial for unlocking the full potential of machine learning in driving business efficiency and success in today's data-driven world. Stay ahead of the curve and leverage the power of MLOps for optimal business outcomes.
Comments